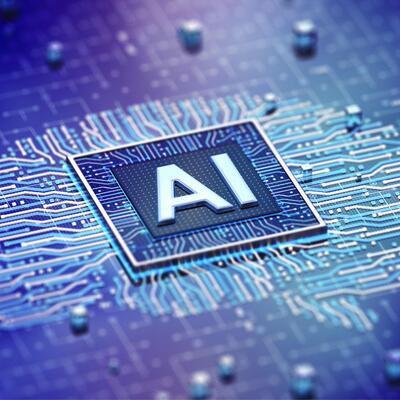
Artificial Intelligence, Real Climate Impacts
Guests
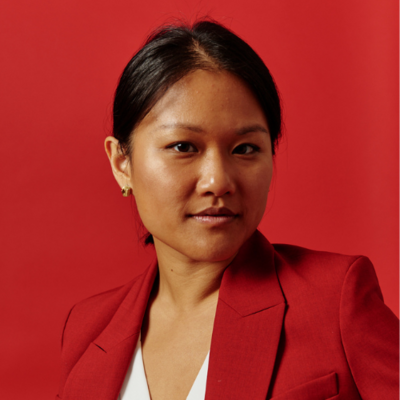
Karen Hao
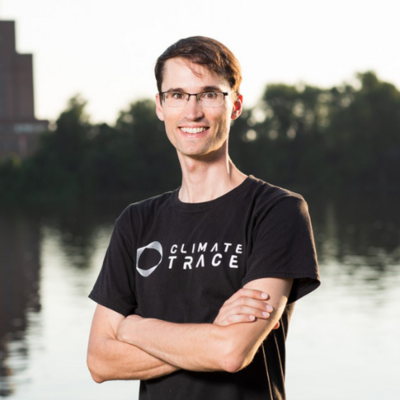
Gavin McCormick
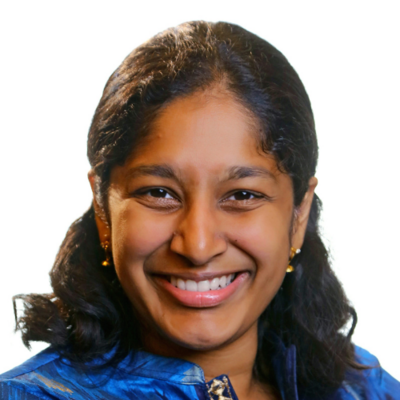
Priya Donti
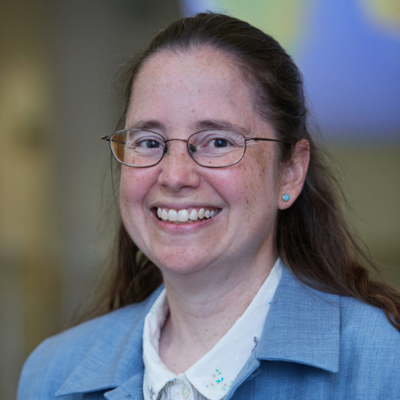
Amy McGovern
Summary
Artificial intelligence can do some pretty amazing things, including for the climate. But, as with most technology, there are significant trade offs. The energy used by AI is massive and growing.
Tech giants like Microsoft, Google and Amazon are building enormous data centers to make AI possible. Karen Hao, a contributing writer for the Atlantic who also has an engineering degree from MIT, visited one of these data centers in Arizona. It was a 97 degree day, and the data center stretched on into the desert. Hao decided she would walk around it to get a visceral idea of how big it is. She says, “Within two legs of the rectangle. I just started feeling very, very heat exhausted and I'd run out of water. It had already taken me around 20, 25 minutes and I was like, I gotta get out of here.” Companies are making huge investments in giant data centers. Hao says Microsoft alone is spending around 10 billion a quarter now on data centers.
Most of the hype right now is around generative AI. Think: ChatGPT. As a matter of fact, the G in ChatGPT stands for generative. The basic idea is that AI is being fed our data to train models that generate more data like that. Karen Hao says, “It's taking our writing to generate more writing. It's taking our images to generate more images.”
But not all flavors of AI use the same amount of energy. Much of theAI that might benefit the reasons is referred to as predictive AI. Predictive AI tends to use existing data to help it make predictions, rather than generating new sentences or images the way generative AI does. For example: it might use our images to make a prediction about what's in another image. Hao says, “Like cancer detection systems or facial recognition systems.” And predictive AI uses far less energy. This is because predictive AI is trained on a specific task, and once it achieves the desired accuracy, its energy use falls dramatically.
Predictive AI is also being used to track emissions. Climate TRACE, an independent greenhouse gas emissions tracker backed by former Vice President Al Gore, is one such organization. Gavin McCormick, Co-Founder of Climate TRACE, says, “we can see that some steel facilities pollute about 10 times more emissions than others to produce the same product.” That data helped companies like GM and Tesla switch to steel factories that produced less emissions. McCormick says, “Our hope is that this is a way that data can make it kind of painless to reduce emissions.”
“AI is being used in all sorts of ways to facilitate climate action from things like helping us better forecast solar power on electric power grids in order to help us balance grids with large amounts of renewables,” says Priya Donti, Assistant Professor at MIT and Co-founder and Chair of Climate Change AI. Efficiency is one of the best ways to reduce carbon pollution. If we didn't need so much power, we wouldn't need to burn so much fossil fuel. With more efficiency we could switch to renewable energy more quickly. AI can help do that, even with simple tasks like optimizing heating and cooling systems in homes and buildings to save energy.
Nowcasting is a weather forecasting model that combines a description of the current state of the atmosphere and a short-term forecast. Amy McGovern, Professor of Computer Science at the University of Oklahoma, says, “our current average [nowcasting] warning is about 15 minutes. Can you imagine if you could bring that up to 30 minutes or 60 minutes?” McGovern also says, “As our climate is changing, a lot of these extreme weather events are changing. And I think AI can be used to help us improve our prediction and understanding of these events and, and be able to weather them better.”
Episode Highlights
3:53 - Karen Hao on visiting a data center in Arizona
7:40 - Karen Hao on the main categories of AI
10:26 - Karen Hao on the energy usage of different types of AI
18:34 - Gavin McCormick on the state of emission tracking with the help of AI
31:19 - Gavin McCormick on the energy consumption of AI
35:13 - Priya Donti on using AI to help optimize the grid
42:44 - Priya Donti on the pros and cons of AI
44:46 - Amy McGovern on using AI to help predict weather
52:21 - Amy McGovern on the future of AI and weather
What Can I Do?
Resources From This Episode (4)
Full Transcript
Note: Transcripts are generated using a combination of automated software and human transcribers and may contain errors. Please check the actual audio before quoting it.
Male AI Voice: I’m not Greg Dalton.
Female AI Voice: And I’m not Ariana Brocious.
Male AI Voice: But this is Climate One
Female AI Voice: Today’s episode is all about artificial intelligence and how it’s going to solve the climate crisis.
Male AI Voice: That’s right — how harnessing the amazing power of AI will lead all of humanity into a greener, cleaner world.
[music change ]
Ariana Brocious: Whow, it's so strange. The intonation is totally wrong.
Greg Dalton: What is that thing? That didn't sound like me.
Ariana Brocious: Also, that's not how we feel.
Greg Dalton: No, I'm not buying that hype at all of, you know, the AI is going to do wonderful things in the Bay Area. That AI hype is just everywhere.
Ariana Brocious: And yeah, there is a lot of hype. There's a lot of hype around what AI can do, right? Artificial intelligence has a lot of powerful applications and we're starting to see some of those right now.
Greg Dalton: A lot of hype and a lot of fear, particularly around computers taking jobs from knowledge workers. A lot of technological displacement has been factory workers, blue collar jobs. Now it's coming for us, white collar workers.
Ariana Brocious: Yeah, it is. It's concerning for a lot of people, though. I will say selfishly, from what we just heard, I think that radio host jobs are safe for the time being, Greg. I think we still sound pretty human, so that's good.
Greg Dalton: Maybe true for me for however many working years I have. For younger people like you, I'm less sure. My bigger worries are around AI's impacts on the climate. No, I do care about your career.
Ariana Brocious: Thanks, Greg. Yeah, there are climate impacts and they cut both ways. And as we'll hear on the show today, AI can help optimize the electric grid, make heating and cooling buildings more efficient, and do really cool things like detect where emissions are coming from. And it's able to complete these assessments in a fraction of the time it would take for a normal human.
Greg Dalton: Like most things in life, there are trade offs. The energy use of AI is massive and growing. A recent study estimates that in just a few years The extra energy need will equal whole countries the size of Sweden or Argentina.
Ariana Brocious: That's unbelievable. That is a lot of power.
Greg Dalton: I'm personally terrified that AI's insatiable power demand will more than outweigh any societal benefits.
Ariana Brocious: And that's the central question of today's episode. How do we weigh AI's environmental costs versus benefits?
Greg Dalton: And it's a complex topic we'll do our best to unpack for you today.
Ariana Brocious: So we're going to start with the fact that not all AI is created equal. There are different kinds of AI tailored for different goals.
Greg Dalton: And before we started working on this, I lumped all AI together. I thought AI was like Siri or ChatGPT, where you put in a question and then AI gives you an answer in written or spoken form.
Ariana Brocious: Yeah, it's still a really new field for most of us, and we're just kind of getting up to speed. ChatGPT uses what's called generative AI, and that's actually what the G stands for. And GPT stands for, so it's supposed to generate new sentences or new images. And this is what has my journalist and copywriter friends really scared, right? Because it's kind of coming for their, their jobs, their work. There's also predictive AI, which uses existing data to do things like predict the weather or detect cancer in a mammogram.
Greg Dalton: Predictive AI uses far less energy than generative AI, and it's also probably where the most useful climate applications are.
Ariana Brocious: Right, you can think about the climate applications of better knowing the weather, right? That's going to be increasingly useful in the years ahead. So this balance of whether AI will be good or bad for the climate will probably depend on what kind of AI we're talking about and how it's being used.
Greg Dalton: To help us get a better idea of the overall AI picture, We want to start today's episode with Karen Howe. She's a contributing writer for the Atlantic who also has an engineering degree from MIT. These days, her writing focuses on the intersection of AI and society, and her reporting has taken her deep into the heart of artificial intelligence, including to the huge computing facilities that make it run.
Karen Hao: I'd never been to a data center before, um, and I really wanted to see it in person. And right before I went, I actually was speaking with a scholar, Mel Hogan, who had, has been writing about data centers for a long time. 10 years and I was asking her, Oh, like how big is the data center going to be? Like, what should I be expecting? And she was like, Oh, when I first started writing about data centers, I used football fields as a comparison. and now football fields don't make sense anymore. Now it's like university campuses.
Greg Dalton: Wow.
Karen Hao:and so when I arrived at the corner of this data center campus, it was like, I was in the middle of this really kind of desolate area within Arizona where there's farm fields on one side and then there's kind of just desert land, for miles and miles and miles. and when I stepped out, it was like 97 degrees and it was, that was actually the coldest, coldest day of that week. and the first thing that I thought was, well, let me try walking around the status center just to get a bodily sense of how big this is, because I could see it stretching out further than I could see. But I couldn't really, you know, when you're in a place that there's not really a lot of landmarks, you can't really assess like, how large is this space that you're in? So I just started walking and thinking, Okay, I'm just going to time myself and see how long it takes me to walk around the whole thing. And it did not work. Within,two legs of the, of the, um, rectangle, I just started feeling very, very heat exhausted, and I'd run out of water. It had already taken me around 20, 25 minutes, and I was like, I gotta get out of here. I'm gonna pass out if I don't leave. And it was, it was a really stark kind of illustration of how big these things are and also where we're actually building these giant silicon monstrosities. Like we are choosing quite intense, hot, dry areas to build these things.
Greg Dalton: So I got, I got to ask, why did they build this thing in the Arizona desert?
Karen Hao: So Arizona has actually become a really popular place for data centers. and part of the reason is because of its proximity to California. It also has cheap land. It has very importantly, a large enough utility company, to provide the type of infrastructure that you need to deliver the energy density, to a data center. And also it doesn't have earthquakes, it doesn't have floods. Like Arizona, other than the heat, has a lot of other things going for it. But of course the heat and the lack of water, and the sheer amount of energy that data centers are now requiring also makes Arizona sort of a controversial. place to have something like this.
Greg Dalton: And you've written that Microsoft alone is on pace to build between 50 and 100 new data centers each year for the foreseeable future. How much of that growth is due to AI?
Karen Hao: A significant amount of that growth is due to AI. Of course, we can't fully ascertain because these companies, and Microsoft in particular, does not actually have any transparency into the amount of AI growth that they're experiencing and what share of their data center growth is driving or supporting the AI development and AI use, but you can just see kind of from the historical patterns for Microsoft that once they made this all encompassing commitment to support it. Open AI, which is the main driver of the AI revolution right now, the amount that Microsoft has been spending on data centers has dramatically increased. So they're spending around 10 billion a quarter now.
Greg Dalton: So AI is not just one thing, yet people talk about it as it is. You know, how do you define different, the main categories of AI?
Karen Hao: Yeah, this is a great point. AI has many different technologies. and right now we're seeing this huge wave of what we're calling generative AI technologies. And that, generative AI, you can boil it down to the idea that it's just taking a lot of our data, to train models that generate more data like that. So it's taking our writing to generate more writing. It's taking our images to generate more images. But before that, there were what we now call predictive AI technologies, which is just taking our data to then make predictions about the world. so it might take our images to make a prediction about what is in another image or what people might may have heard of before is things like cancer detection systems or facial recognition systems. These are all of the category of predictive AI, where you're just trying to identify and classify something in the world.
Greg Dalton: And is there a meaningful difference in energy consumption between generative and predictive AI?
Karen Hao: The way that AI is being developed has completely changed. So before, when you're talking about predictive AI, you start with a problem, like, I want to detect cancer in this MRI scan, and then you collect data that's very well scoped to solve that particular problem. You train an AI model to do that specific thing, and you measure it on a benchmark that is to measure that performance. So you, you will ultimately do all the work and then you might develop an AI system that can do 99% accuracy on identifying breast cancer in an MRI scan, for example. and because it is like very narrowly and well scoped as a problem, the energy consumption is significantly less than what we're doing now. Now with generative ai. when you try to teach a system to be able to generate. any kind of writing or any kind of image, the sheer amount of data that you are feeding into these systems has already dramatically increased orders of magnitude. and we see stories about how these companies are running out of data on the internet to consume because of the sheer data imperative of these technologies. And once you have that much data, you also have a far larger AI model. And that means the amount of energy that goes into training it and also running it after it's finished developing has dramatically increased multiple fold.
Greg Dalton: And you, you said that Microsoft has been the primary vector for conflating the kind of AI that can be good for climate solutions, like optimizing the grid, maybe predicting weather, with the type of AI that like chat GPT is the greater villain when it comes to energy use, as you've just been describing. what do you think about Microsoft conflating the different types of, you know, sort of good energy, good AI and energy, bad AI?
Karen Hao: Yeah, I think what Microsoft has done that's quite clever, I guess you could say, is they Talk about AI as though it's one thing and they'll go to these, these forums like the World Economic Forum or COP, and they'll say, it is critical to accelerate the advancement of AI to combat climate change to make sure that we attain sustainability. And if you go into the weeds, what they're talking about, is there are many types of predictive AI technologies that are in fact great at optimizing, um, the grid to make sure that as more electric vehicles plug into the grid, you actually can distribute the power in a way that doesn't affect the, the grid resilience too much or they're talking about optimizing the energy consumption of a building to make sure that it drops by 40 or even 60%. But when they say accelerating the advancement of AI, what people register in the public is they think that they're talking about something like ChatGPT, because that is what Microsoft is supporting the development of right now, and so they think, oh, we need more ChatGPTs, we need more generative AI, we really need to double down on this in order to solve climate change. And that's actually not at all, they are not talking about the same thing. They're talking about a fundamentally different category of AI technologies that might be helpful. And they're using that as a cover to then allow or excuse the kind of investments that they are pumping into a much more energy consumptive version of AI.
Greg Dalton: I usually think I've thought about Microsoft as a climate leader. They were early to have an internal carbon price. They used to have people track the flights they took. They said they were going to zero out the carbon emissions of the entire history of the company. I think Microsoft's been a pretty legit climate leader. Is the pursuit of AI putting that at risk?
Karen Hao: That is absolutely what I heard from Microsoft employees, both current and former. There are a lot of employees that are very deeply concerned from what they're seeing within the company, and they think that there was a time when Microsoft was taking remarkable strides and pushing the industry forward, in terms of sustainability commitments, but that now, the imperative to support this AI development, because it is just so profitable and it's done wonders for Microsoft's share price, is starting to consume the original commitments in sustainability.
Greg Dalton: Right. And you also quote Sam Altman, who said at Davos that quote, we still don't appreciate the energy needs of this technology, end quote, and he then goes on to seem to suggest that we need an energy breakthrough such as fusion. So we're building these things that are going to need this energy supply that doesn't yet exist. We're creating these. I'm just getting all worked up here talking with you about how we're creating these beasts and the implications of what's being created and how it seemed to be that the seduction of AI and the profits is taking Microsoft off course of its sustainability commitments,
Karen Hao: And not just Microsoft, I think the entire tech industry, Google, Amazon, all of these companies also made sustainability commitments at one point. and that also did wonders for their share price. They were rewarded for that in financial markets for making these really strong commitments and they were rewarded in their brand, for making these strong commitments. And now all of them are in this race to the bottom to basically lay down as many data centers as they can to support, a technology, a version of AI, generative AI, that still has not fully demonstrated clear value to people, proportional to the amount of resources that it uses.
Greg Dalton: Right. Well, we've lots of hype cycles from Silicon Valley. They don't pan out the way the tech bros promise. Um, so. Do you ever get angry reporting on this?
Karen Hao: yeah, whenever I, I mean, recently, there was this article from The Information scooping the fact that Microsoft is sketching out plans to build a data center that would cost 100 billion, just a single data center that would cost 100 billion, and it would be the most expensive, and the largest and the most powerful data center that has ever been built in the history of humanity. And just seeing this kind of headline, it, it make, it does make me angry because I'm like, what are we doing here? Have, has no one noticed what we are allowing these companies to do? Are we just going to continue sleepwalking into this future where we end up with no energy or we end up consuming more and more and more and more fossil fuels into our oblivion? And whenever I, I get angry, my husband's always like, take that energy, convert it, and write it into your next story. Like, keep doing what you're doing. And, um, and that's what keeps me motivated. But it is, it can be kind of disheartening and demoralizing because sometimes I'm writing these stories and I'm like, is anyone paying attention? Like, we are it. in a massive planetary crisis right now. And yet we're still just talking about, ooh, like a hundred billion dollar data center. Wow. So powerful. So cool. Like what, where? Yeah. It's just, it's like really unfathomable to me.
Greg Dalton: So, not all flavors of AI are the same. Generative AI, like ChatGPT, uses a lot more energy than predictive AI, which is trying to answer more specific questions with smaller sets of data. How do you personally balance AI's potential for reducing the climate crisis with its potential to make it a whole lot worse?
Karen Hao: There are so many AI technologies that we already have that could improve things from an energy and climate perspective, like we already have algorithms that reduce the energy consumption of a building and optimize it, or we already have technologies that can optimize the grid to bring more renewables onto the grid. We should just be more clear eyed about the differences between the different AI technologies and where we should be applying what.
Greg Dalton: Mm hmm. Karen Howe, thank you so much for sharing your insights with us on Climate One.
Karen Hao: Thank you so much for having me.
Greg Dalton: Coming up: Researchers are using AI to help track emissions, and to see who is being dishonest about their pollution …
CLIP: The type of misinformation we are actually seeing is typically corporate.
A really disproportionate share comes from oil and gas companies.
Greg Dalton: How watchdogs can use AI. That’s up next, when Climate One continues.
Ariana Brocious Please help us get people talking more about climate by sharing this episode with a friend. And we’d love to know what you think of the show. Please give us a rating or review. You can do it right now on your device – and it really helps people find the show. Thanks!
Ariana Brocious: This is Climate One. I'm Arianna Brocious. AI use is growing and growing. That means it needs more energy, more resources, and produces more emissions. Yet, it's also being used to track emissions. One of the groups using AI in this way is called Climate Trace. It's an independent greenhouse gas emissions tracker backed by former Vice President Al Gore. Their data is available to everyone for free, and the results they've published in just the last few years have created a whole new level of staggering transparency. Polluters who are able to mask their emissions through their own reporting or misinformation campaigns can no longer lie and hide. And even honest players have more accountability tools at their disposal. Gavin McCormick is co-founder of Climate Trace. I asked him how AI is making emissions tracking on a large scale possible, and what results he's seeing.
Gavin McCormick: One of the things that has happened for a long time in climate change is we've had good monitoring of the total emissions in the atmosphere. We've had pretty good monitoring at a country level of what's going on, but the difference between what's the total industrial output of China versus what is this factory's carbon footprint, is it's way more actionable. So just to give you one among many examples, we've been working with a bunch of companies who buy a lot of steel and we can see that some steel facilities pollute about 10 times more emissions than others to produce the same product. So now that we have data like this, we've been able to help a lot of companies like Tesla and GM, start to buy steel from steel factories that were really clean, but actually had more capacity. And our hope is that this is a way that data can make it kind of painless to reduce emissions because nobody had to go build a new clean steel factory to get more steel. We're just using what we already have smarter.
Ariana Brocious: So shifting the sourcing to a source that is less greenhouse gas intensive, uh, or emitting. I was recently at an oil and gas conference and there was a lot of discussion about methane emissions in particular, and the rise of more monitoring like climate trace. And, my guess is that big industries are concerned and aware that they're now going to be monitored more closely. And so they may need to rein in some of their practices. But when we talked before, you also mentioned that there are just sort of unknown emissions, even by companies or, um, practices that may be trying to kind of keep their emissions in check. So tell me a bit about what you found when you've been able to point source these emissions.
Gavin McCormick: Yeah, one of my favorite examples is landfills. So landfills turn out to be a surprisingly significant source of emissions. The biggest thing we've found using satellites to look from space is there's just a lot more landfills worldwide than were commonly known. So we've compared to a lot of nations inventories of landfills, and in some cases there's four times more than were known. What I think is interesting about landfills, it's not like anyone was trying to hide those emissions. They were just kind of forgotten. And so I think one of the most interesting things data does is it doesn't just answer the question that you thought to ask, it sort of says, what emissions are you maybe not paying attention to that, uh, maybe you're not opposed to doing better.
Ariana Brocious: So that's great. That's a great example. And then let's return to this idea of the sort of keeping people honest, you know, for lack of a better word. So how has the data that Climate Trace has been gathering helped, industries or countries maybe from a regulatory side, be able to better track and monitor emissions.
Gavin McCormick: Yeah, so, we've worked, for example, with one country that did not know how, uh, intensive its own oil and gas sector was in terms of emissions. I can't promise that the next step is they're going to clean it up, but I can tell you that the regulators literally had no idea, and we were able to quietly, behind the scenes, inform them that there's a lot more methane coming out of their facilities than they thought. We worked with another country, who, uh, suspected that the states of that country were lying to the federal government about their own emissions, and the federal government was interested in cracking down on emissions, and what we were able to do was give them sort of objective third party validation to which of their states were probably giving them accurate data, and which of their states maybe were fudging some numbers. We will never know behind the scenes what the next step is, but we can tell you that a year later that country announced some pretty significant environmental policies, and we like to think we might have had a hand in that.
Ariana Brocious: Cool. Okay. So landfills, as you were talking about, are a huge source of methane. Another big source is feedlots and dairies, particularly here in the US. There's a lot. When we look at a country like the US and these sources, are there any kind of national inventories of emissions data for those industries that this is complementing, checking, and so forth?
Gavin McCormick: Yeah, it's a mix. So we've really seen different countries are in different places. So, for example, for factory farms, the country worldwide that does it best is Uruguay. They have a really, really good inventory of every cow in the country. A country that does it really badly is the United States. Where, uh, for political reasons, uh, there's been a lot of pressure to actually forbid the government from keeping, the federal government from keeping a detailed inventory of factory farms and their emissions. That's fairly complicated. But the United States actually is quite good at monitoring, uh, transparently power plants, which other countries don't do very well. And we see patterns like that all the time.
Ariana Brocious: So you're saying, just to underscore this, that the US prohibits the collection of emissions data from factory farms, from large dairies and, and feedlots.
Gavin McCormick: The EPA is not allowed to have a list, and that is clearly not a scientific, that is clearly a political decision.
Ariana Brocious: Yeah, interesting. Okay. so. This episode is about the role of artificial intelligence. Climate Trace went from publishing data about thousands of emitters to 350 million emitters in just over a year. How did AI enable that?
Gavin McCormick: So AI, and really all software is like this, but AI is a good example, really has this property where it can take a long time to make a model, but then running that model many, many times is actually quite easy. So it took us more years to set up the ability to monitor a facility from space. Then it took us to go towards more than half of global facilities, and we're hoping this year to more or less finish the job.
Ariana Brocious: So we've talked on this show about the energy demands of AI, and the concerns around how much it can consume. And when we talked before, you were very helpful in sort of explaining that there are differences in where the power is coming from, how clean it might be at any given time. and then also the sort of power needs of different aspects of AI implementation. So you were just describing building the tool. And from my limited understanding, that takes a lot of power, right? Whereas maybe running it doesn't. So could you unpack that a bit, the sort of complexities behind the concern around AI's energy usage?
Gavin McCormick: Yeah. So first of all, I think that energy usage is the environmental issue here. We as a society are using so much more AI now. All signs are pointing towards more and more. And it's really that electricity consumption that, uh, could cause such environmental harm. And in general, the pattern of AI is training a model is very, very electricity intensive, whereas using a model, not necessary, very electricity intensive. Kind of similar to building a factory is harder than using a factory. and so what I think is really interesting there is that, uh, that means that in many cases you have an enormous amount of electricity consumption at a particular time. And then kind of like a little dribble of electricity consumption later. And, the carbon footprint of that totally depends on, well, what time was it? Was it a time when the solar panels were shining and the wind was blowing and there were relatively clean power plants on the grid? Or was it a time when it was peak energy demand and they were running extra oil plants and coal plants to run that power? You could have a radically different carbon footprint for the exact same AI model training, um, which is kind of a new thing. We're not used to thinking about electricity that way as a society.
Ariana Brocious: Yeah. So you're a co-founder of climate trace, which is mostly what we've been talking about. You're also co-founder and executive director of Watt Tme, which is a separate nonprofit that helps people in companies and governments slash their emissions. So tell me how the work of Watt Time can address some of this, you know, less carbon intensive energy sourcing on the grid.
Gavin McCormick: Yeah, so we're a non profit trying to raise awareness at this point that, um, Watt time you use electricity really affects its carbon footprint. And so a great example of that is, if you have data, well, when was the wind blowing? When was the sun shining? What is the moment that they were throwing away surplus renewable energy because nobody was using it? And if you train your AI models at that time so much cleaner, then you can Then running the exact same model at another time, and it's actually the same way that you reduce the carbon footprint of charging an electric vehicle, charging a battery, running a thermostat. But what's particularly interesting about AI is so much of the electricity consumption happens in a really short time, and you can even do crazy things like you could even choose to, you know, train a model in a different location. So, we're also working with companies like Microsoft to say, hey, if it's a really clean grid in France right now, and a really dirty grid in Germany right now, uh, maybe you want to run that compute job in France today. And that's a very different way of thinking about energy than we're used to.
Ariana Brocious: This sort of gets at this like meta idea of, you know, AI being used to make AI better. Um, is that in practice happening? Are we seeing AI being implemented to make itself cleaner?
Gavin McCormick: Yeah. We are now using A. I. to figure out how to run A. I. to have a lower carbon footprint. and there's this wonderful, eat your own dog food component of it.
Ariana Brocious: Yeah. As we know, there's been commitments made by countries all around the world to reduce their emissions by certain targets to hope to meet the Paris Accord goals of keeping global warming under 1. 5 degrees. how are governments using Climate TRACE’s information so far?
Gavin McCormick: Yeah, the main thing that we've heard so far is it is difficult to affect the official system of country. There's often years of inertia in the ways that the books are kept, but a lot of what actually happens in the Paris agreement is about reputation. So the secretary general of the UN in particular has been very insistent that having an open transparent data set like Climate TRACE that makes it really clear which countries are lying about what really changes the politics in a hurry. One thing I'm delighted to share is that the outcome of that has been different than we expected. So we went into this thinking that we were going to find a bunch of liars and that there was going to be really awkward conversations at the next COP. That's really not what happened. So one of our biggest findings is that the vast majority of countries have been much more honest with each other. Then the climate negotiators themselves thought. And so oddly, the political implications have been a higher level of trust between countries trying to figure out whether they want to kind of like, uh, trust each other more and commit to deeper cuts, which is not the way we expected this project to go, but that's science.
Ariana Brocious: That's great. I mean, that's, uh, yeah, counterintuitive, but, nice to hear that the countries are sort of, being honest about what they're doing. Yeah.
Gavin McCormick: Yeah, you know, trust is the foundation of the Paris Agreement. I think it's easy to, a lot of folks kind of think of it as like, there's some set of rules or some cop who's going to force us to cut emissions. There's nothing like that. Yeah. Yeah. The only thing the U.N. has is reputation and trust, and it turns out if we have that, that really matters.
Ariana Brocious: hmm. So we touched on the concerns around energy consumption of AI. There are some other concerns as well. One of those is misinformation. So my brain goes to things that got a lot of attention, you know, like videos that have been edited, um, to make somebody sound different or to say something different than they actually did, um, or images that have been altered to misrepresent something. But when we're speaking about misinformation, particularly in the climate space that is aided by AI, what does that look like?
Gavin McCormick: In this case, I actually think that AI is more of a solution than a problem. I think in the specific space of climate, we've been up against misinformation for a long time. This goes back to the 70s. And the general playbook is just kind of obfuscate, deny, pretend nothing's happening. And AI that can just show you real imagery, not tampered with, of what actually happened. It really tends to empower those seeking truth more than those seeking, um, the opposite. And so as a general rule, I'm actually not terribly worried about misinformation from AI in the climate space, although it probably is a big problem in other fields.
Ariana Brocious: So you, you feel that way because you think that the power of AI, it can, validate truthful data,
Gavin McCormick: Yeah. And I think one of the things that is really interesting is the only place I am seeing some misinformation is As it gets harder and harder to deny the real world physical emissions, we're seeing more corporate shell games on saying, Ah, those emissions might be happening in the real world, but due to some funny accounting rule, they're not my fault. They're not my problem.
Ariana Brocious: or I've offset them over here.
Gavin McCormick: or I've offset them over here. My favorite new trick is one company is trying to cite data centers, the ones powering AI near nuclear power plants or windmills because they argue that that makes them clean. That doesn't do anything for the windmill or the nuclear power plant.
Ariana Brocious: Right, right, right. Unless they're connected directly to it, which they probably are not.
Gavin McCormick: Right. Which they never are.
Ariana Brocious: Well, speaking of the energy consumption, How concerned are you about the actual amount of energy that AI is going to continue to consume?
Gavin McCormick: Yeah. So this is another area where trying to follow the data, I reached a conclusion that is not what I originally would have expected. So I think the volume is going to be large, but also I am really seeing serious activity by most AI companies to, to really try to power it with renewable energy and it helps the renewable energy so much cheaper now. So I actually think as a society we are doing pretty well on the carbon from AI and people actually maybe should be relaxing about it a little bit. I do think that other industries like Bitcoin are very different. We've noticed a real difference in, you know, if Bitcoin and AI both use a bunch of electricity, how much are different companies trying to power it from clean sources or not? It's Bitcoin where I'm seeing more powering it on coal and oil, and in AI I'm seeing a lot of powering it on solar and wind.
Ariana Brocious: Interesting. Well, and also with Bitcoin, it's sort of in an exponentially more energy needed to crack the code, right? As it, I don't think AI works quite the same way. So, how do you personally balance AI's potential for mitigating the climate crisis with some of these risks we've talked about?
Gavin McCormick: Yeah, so we actually just, last week, tried the exercise of counting our own carbon footprint. It turned out that, we can measure about 50 million tons of emissions that we've reduced and something like three tons of emissions that our AI carbon footprint has caused. So it's not zero, but that ratio is pretty staggering. so we actually concluded that flying is a bigger source of carbon footprints for our team than all the AI compute we use. And, I don't mean to say we shouldn't be cleaning up AI, but I do think that, when you actually look at the numbers, particularly given how much of it is powered on clean energy, it's probably not as bad as you think.
Ariana Brocious: And then hopefully there's this potential for continued optimization of these other renewable energy systems that we want, to actually improve the grid and emissions writ large, right?
Gavin McCormick: Yeah, and so, you know, a different category is AI is also very much used by the coal industry and the oil industry. And what generally happens is that doesn't get talked about, whereas the AI power of the wind industry gets talked about. So I couldn't tell you how many extra tons of pollutions are we going to have because the oil industry has better technology now, but that's probably a large number too.
Ariana Brocious: Mm hmm. Yeah, that's a good point. Gavin McCormick is co-founder and executive director of WattTime and co-founder of Climate TRACE. Gavin, thanks so much for joining us on Climate One.
Gavin McCormick: Thanks so much for having me, this was fun.
Greg Dalton: You're listening to a conversation about the climate implications of artificial intelligence. Coming up, we know that burning fossil fuels continues to amplify fires, floods and droughts. Can AI help protect us?
Amy McGovern: A lot of these extreme weather events are changing, and I think AI can be used to help us improve our prediction and understanding of these events and be able to weather them better.
Greg Dalton: That’s up next, when Climate One continues.
Ariana Brocious: This is Climate One. I'm Arianna Brocious. One of the best ways to reduce planet warming emissions is through efficiency, basically using less energy. If we didn't need so much power, we wouldn't need to burn so much fossil fuel. And predictive AI can help us understand how to do that. Priya Donti is an assistant professor at MIT and co-founder and chair of Climate Change AI. A global nonprofit trying to foster the responsible use of AI for impactful climate action. Her own research focuses on optimizing the country's electric grid and maximizing efficiency throughout our energy system. She sees countless ways AI is already being used to advance the cause.
Priya Donti: AI is being used in all sorts of ways to facilitate climate action, from things like helping us better forecast solar power on electric power grids in order to help us balance grids with large amounts of renewables, to helping us better optimize things like heating and cooling systems in buildings in order to improve their efficiency by taking into account things like the occupancy of the building, the temperature of the building, in order to improve things like the, thermal comfort of the building while still reducing the overall energy use, to helping us do things like accelerate the discovery of next generation clean technologies like batteries by helping us to analyze the outcomes of past experiments and then suggest which experiments we should try next, but also helping us adapt to the effects of a changing climate through things like extreme event forecasting, through flood forecasting and flood mapping, as well as strengthening things like, policy, education, and finance by helping us get more fine grained information to provide an input to those kinds of strategies.
Ariana Brocious: So one area I'm really fascinated by that I know you work in is grid optimization. Specifically looking at that area. Can you tell us a bit more how AI is being used?
Priya Donti: When we think about our power grid, it's the system that needs to be maintained at this sort of exact balance between supply and demand at every single moment in time. And so AI is being used in a couple of ways across this. So one, is sort of helping us to forecast things like solar power or electricity demand. So in the case of solar power, for instance, what you would do is you say, I might have some historical data about how much solar power was produced. And related to that is information about things like how sunny was it? Were there clouds overhead? And I can basically take in the same kind of information. Maybe I have a forecast of how sunny it'll be. Maybe I have a video of how clouds are moving overhead. And I can try to learn patterns in that underlying data to figure out, in the past, how did it relate to how much solar power I actually got, and then use that same kind of relationship between the data to make predictions in the future of if the cloud cover looks a certain way and the sun looks a certain way, what will my solar power output look like?
Ariana Brocious: So that makes total sense to me. I'm also thinking about applications of balancing that grid, And that is currently handled by humans, right? With the help of computers and software. But is there a significant leap forward that can be made with artificial intelligence in this space? Or is it just kind of making better what we already do?
Priya Donti: Yeah, So right now what tends to happen is that a power system operator has sort of a view of what the power generators are on the system as well as some aggregated notion of what demand will be, and as well as some notion of what the solar power production will be and the wind power production will be. And then they figure out how to kind of control your controllable power generators, like your coal plants, your natural gas plants, your nuclear plants, in order to balance the overall system. And this is done, as you said, by humans who are aided by computers. So there's some optimization algorithm that is running, that's actually taking in information about the system and its physics and the costs of different power generators, and actually spitting out a suggestion of how you should actually schedule your power generators. And then that might be modified by humans based on knowledge they might have that's not in the system, or based on considerations like, okay, 24 hours ahead, there's a different person at a different desk who thinks about one hour ahead, and so you need to coordinate with each other. and there are a couple of ways that this kind of needs to change going forward. So one is that the computational tools that power grid operators are using today, they are not scaling to meet some of the kind of requirements that we have for managing a grid that needs to be managed faster because you have more and more variability from things like renewables and also at larger scale because you have more devices, more batteries, more electric vehicles, more things that are plugged into the grid that you have to account for. and there are ways that AI and machine learning can help to speed up some of the algorithms behind some of those computational tools in order to enable them to run faster and deal with a larger scale system.
Ariana Brocious: So there's a lot of discussion right now about AI. It's kind of viewed as this next big thing. There's excitement. There's also a lot of trepidation around how much energy it uses or can use. And I'm wondering how much you personally worry about the energy demands of AI.
Priya Donti: Yeah. So I definitely worry about this, and not necessarily because I necessarily know that the impact is going to be big or small. But precisely because we don't know, and as a result, we actually don't, I think, have as good a sense of what specific levers we can really be taking in order to address some of the growing computational impacts. I mean, fundamentally, we have to drive every single sector. to net zero when it comes to greenhouse gas emissions. That includes AI, and so we need to know what's going on underlying in terms of how compute loads are being run and things like that in order to do something about it.
Ariana Brocious: Another aspect of the potential downsides of AI revolve around its use in misinformation, particularly in the climate space. And I'm curious what your thoughts are there. And if you've seen any positive developments in, um, maybe limiting the spread of, of AI generated climate misinformation.
Priya Donti: Yeah, climate misinformation is something that I, again, really do worry about. I mean, climate misinformation and sort of misinformation in general, its effects on how we relate to each other, how we relate to facts, and that the broader implications of that for not just climate action, but many other kind of aspects across society. so I think this is another example of a place where you have an algorithm that's embedded in some broader context. So, for example, Often social media platforms are optimizing for clicks or engagement, and incendiary content tends to be more engaging in various ways, and so the algorithms underlying learn that. It's like, okay, you're optimizing for engagement, so what is more engaging? And you kind of tend to trend towards more incendiary or potentially false content. And so that's, I think, really concerning, but something that sort of, in theory, a change in the thing you're optimizing for can help with. There are some ways that AI is being used, I think, to, to positively help with this issue. So things like, um, AI is used for, in some cases, misinformation detection. and then as a result if you detect something that might be misinformation that gives you the opportunity to do something like annotate it and kind of display that information to people. And so I think there are some good applications here but I think fundamentally without changing the objectives of the platforms that AI algorithms are accelerating, that misinformation is going to be a huge issue and changing objectives, in a situation where engagement, is ads, is money, and that's how these companies are profiting. You really have to change the underlying incentives in order to really change what's going on here.
Ariana Brocious: Tricky. So overall, how do you balance the potential risks of applying artificial intelligence to climate challenges with the potential benefits?
Priya Donti: Yeah, so fundamentally, when it comes to AI, there's an extent to which the cat is out of the bag, right? It is used across society, and I think while, I understand the rationale for doing things like saying, let's halt the development of AI, given that it's accelerating systems across society and disproportionately accelerating, again, things for people with money and power already, I do think the cat is out of the bag there. And so then the question is, you know, how do you make sure to sort of steer the way society uses AI in the potentially most beneficial directions? And I think that has to do with a combination of how do you get people who are, first of all, like working in AI. to actually leverage those tools for beneficial causes like climate action and sort of channel that interest, make it an attractive and viable career to be someone who works in climate using those skills. But then making sure that we don't just think of, AI be used for good as layering a couple of AI for good applications on top of business as usual, but we really need to think about how we actually manage our business as usual as well.
Ariana Brocious: Priya Donti is an assistant professor at MIT and co founder and chair of Climate Change AI. Priya, thank you so much for joining us on Climate One.
Priya Donti: Absolutely. Thank you.
Greg Dalton: We know that weather is becoming more and more extreme as the planet heats up. That extreme weather has a real human cost, especially in poor areas. Places that don't have the resources to protect themselves from the worst of the damage. What if AI could help make weather predictions more accurate and make them sooner? That's what I wanted to talk about with Amy McGovern. She's a professor of both computer science and meteorology at the University of Oklahoma. She researches developing trustworthy AI for severe weather.
Amy McGovern: I want AI to be able to make a positive difference in the world. I want it to be able to save lives and save property. And my personal experience with weather isn't just living in Oklahoma, although I think I get every single piece of weather in Oklahoma that I got anywhere else I ever lived. We just get it all at once and sometimes in the same day. And because you experience it every year and particularly every spring, everybody knows that. So, oh, today's the day, you know, and we're, we're worried about the hail. We're all cleaning our garage out. We're getting our shelters clean. We're getting our cars fixed up. We had a storm come through about three years ago, came through town, destroyed all the roofs on the north side of town and all of the cars that were out. Because you're talking about like baseballs that came down and just destroyed all the glass. We're on roof number four in nineteen and a half years.
Greg Dalton: Wow, How is AI improving weather predictions with all this volatility around the country?
Amy McGovern: AI is being used to improve weather predictions. across a wide, wide variety of predictions, right? So you're talking about severe weather and we can talk about that specifically, like trying to improve the predictions multiple days in advance so that we could tell you, you know, eight to 10 days in advance, for example, that there's likely to be severe hail so that you could start to think about your emergency preparedness preparations. Those probably don't matter so much to the individual homeowner, but imagine you're an airline, and you know that, you know, a large chunk of the area where your planes are gonna be is under the place where there might be four inch hail. Planes don't like four inch hail for the record. Um, so you'd like to have them out. And if you have 30 planes parked somewhere overnight and there's likely to be storms coming through, you can't fly them all out in five minutes. That doesn't work that way. So you know, those kinds of operations. We had a discussion with a car manufacturer who was looking to get forecasts both multiple days in advance and then they wanted multiple hours in advance, which is another place AI can help. And then AI can help with the now casting scale, which is trying to help us, you know, our current average warning is about 15 minutes. Can you imagine if you could bring that up to 30 minutes or 60 minutes, um, with a high rate of being right, right? If AI could help you with that, then people could take more protective action. And I think at that scale, you're talking about the individual homeowners. You're talking about the people who say, okay, I have an hour to quickly clean my garage and throw everything from my garage into my house. So my car fits.
Greg Dalton: Right. I get the the time scale longer, better, with high degrees of accuracy to give people more time to prepare, move cars, move planes if you have them. And how about a spatial dimension? Is it really going to affect me in my path? So let's talk about the spatial scale in terms of, you know, my street, my neighborhood, what can you tell me about my risk?
Amy McGovern: Yeah. So the spatial scale tends to correlate with the temporal scale, right? So we're getting a lot broader and the multiple days in advance, you're going to get a really broad spatial scale. Something like the Eastern third of Oklahoma is likely to have these storms and, you know, four or five days in advance. You're likely to get that. The scale that you're talking about at the street level doesn't really exist yet in the sense of we can tell you precisely 30 minutes or 60 minutes in advance that it's going to come down your street. That's just not possible yet. But it will be. and it's certainly a goal that the weather service is working towards. I think the predictability is so low for something like hail and tornadoes that we're not going to be able to get that street level prediction multiple days in advance. It's just not, there's too much chaos in the atmosphere right now.
Greg Dalton: Sure, things are moving around. So expanding the time window, narrowing down the geography in place so I can know about my path, or at least maybe my town, if not my street. And AI has potential to improve these things. I imagine it's an exciting time for you as an AI expert working on meteorology. What gives you lumps in your stomach about AI and weather?
Amy McGovern: I'm worried that. Somebody might misuse it. So AI and weather is looking so promising and it's getting deployed in so many ways and there aren't really safeguards and we don't have any legislation. The EU just passed something called the AI Act that's gonna have a lot of legislation on different AI and it's based on the the risk that you're gonna have. Like they don't even allow things that are unacceptable risk if they're high risk, that get to go through certain amounts of review and things like that. We don't have that in the United States yet. I'm concerned somebody's going to deploy a model. It might not even be weather, but I'm concerned on the weather scale.Imagine that somebody sells you an app on your phone and tells you, this is going to tell you all your warnings and you don't need to worry about it because it's AI and it's going to be right. And then somebody is going to trust it and it's going to be wrong. And I'm not saying AI is always going to be wrong. Just, it's just like any other forecast, right? But it's going to get it wrong sometimes. And then there's going to be a lack of trust, but I also worry that people are going to lose their lives to it, that they put too much trust into it. And I, that worries me.
Greg Dalton: Some studies say that AI's energy use and understand that AI is a broad set of different types of technologies that get lumped together They all require a lot of computer processing power that could take this amount of energy of a country like Sweden, and I hear other talk about AI's potential to solve some really wicked problems. How do your peers and you think about the possible trade off of making climate worse with a bunch of data centers creating emissions while also trying to use the tool to do some good?
Amy McGovern: I think a lot of people just try to stick their head in the sand and pretend that it's not happening. that the cost doesn't exist, but that isn't the right answer. The right answer is to pay attention to the cost and to try not to just train my AI models over and over again. The other answer is to try to look for sustainable data centers. And just trying not to waste cycles. Oh, well, that computing's free. I think for many years people have kind of thought that. It's free. It's free disk space. It's free GPUs, but it's not. It costs energy and people need to realize that.
Greg Dalton: Right?, we don't often think about, all the files we store in the cloud, for example, that's using some data somewhere, right? Using energy. It's not, not free storage in an energy sense or a climate sense. I've read that AI models can be biased. For example, a lot of us have heard about facial recognition and, whether it recognizes primarily white faces, I don't really understand what that means for applying AI to climate problems. Can you talk about how bias could affect climate and weather modeling?
Amy McGovern: Yeah, I'd be happy to. So, It doesn't come into it the same way that it does for the faces that you're talking about, right? Because we're not recognizing white faces and black faces, although that is definitely a problem for AI in general. But if there's an underlying bias in the data set, then the AI models are going to replicate that. That's all they were doing with those face data sets. So if you're trained on only white faces, you just have no clue. concept of what a blackface looks like, because you haven't been trained on it. And the same is true if you're trained on only data from a certain part of the world, So for example, uh, crowdsourced data, anything that's crowdsourced, which means that you've had somebody provide the sensor to you, like the general public, tends to be more clustered in the more affluent areas because it's the people who have the money to buy the crowdsourced, you know, instruments. So if you were trying to do, An air pollution sensor, uh, you know, prediction problem for AI. Maybe you only got your data from the affluent areas because that's where you had the crowdsourced data. That could be a problem. That could create a bias that then your model will then not give you good values for places where there might be really big problems.
Greg Dalton: So how do we build trust into AI in this context?
Amy McGovern: That's a large question. Trust is not a binary, trust is a spectrum, and trust is something that we really need to work with our end users. We need to make sure we're meeting their needs, we need to make sure that they're, you know, seeing what we're doing with the AI that, that they're co developing is the word that, that we're using, that they are part of the development process and that they are putting their thoughts into it so that the AI we develop does meet their needs and that they're evaluating that the AI that you develop and give back and say, here's our model, let's try it. They have feedback that, okay, well that didn't really meet our needs. So let's talk about how we can adjust it. Or here's all the ways in which it's wrong. Let's fix it. You know, things like that. That will really help with trust. and also I don't think you can just develop something and hand it to somebody and say, here's your AI model. You should trust it. That's actually gets us back to the lumps in the stomach question you asked earlier, right? Because I'm going to hand you that model, but it might not work for what you need.
Greg Dalton: Right. So as we wrap up, you know, is there one place you come to about this mix of excitement and, and concern about where AI and weather are going?
Amy McGovern: I'm excited. I mean, you asked me for the concerns, so I gave you some concerns, but I'm excited that AI is going to be really able to improve our predictions, both on the short term and on the longer term, so that we can be better resilient as a species and as an environment, like not just our human species, but all the species. As our climate is changing, a lot of these extreme weather events are changing, and I think AI can be used to help us improve our prediction and understanding of these events and be able to weather them better.
Greg Dalton: Thank you very much, Amy, for sharing your excitement and concern with us candidly appreciate it. Thank you.
Female AI Voice: So, Artificial Greg Dalton, where does this leave you?
Male AI Voice: Well, I see both sides now. AI can be used to solve some tricky problems. But in the process, it may create others. How about you, Artificial Ariana Brocious?
Female AI Voice: I agree. I think the problem is not the computers. It’s how they are used.
Male AI Voice: In other words, it’s the humans.
Female AI Voice: Ha! Ha! Ha! Yes. It’s always the humans.
Male AI Voice: But I still worry about where all my energy is going to come from…
Ariana Brocious: And that’s our show. Thanks for listening. Talking about climate can be hard, and exciting and interesting — AND it’s critical to address the transitions we need to make in all parts of society. Please help us get people talking more about climate by giving us a rating or review. You can do it right now on your device. Or consider joining us on Patreon and supporting the show that way.
Greg Dalton: Brad Marshland is our senior producer; Our managing director is Jenny Park. Ariana Brocious is co-host, editor and producer. Austin Colón is producer and editor. Megan Biscieglia is producer and production manager. Wency Shaida is our development manager, Ben Testani is our communications manager. Jenny Lawton is consulting producer. Our theme music was composed by George Young. Gloria Duffy and Philip Yun are co-CEOs of The Commonwealth Club World Affairs, the nonprofit and nonpartisan forum where our program originates. I’m Greg Dalton.